报告题目:Modern Medical Image Segmentation, AutoML, and Best Practice with MONAI
报告摘要
Nowadays, with technological advancements in algorithm design (such as deep learning) and hardware platforms (such as GPUs), medical image analysis has become a critical step in disease understanding, clinical diagnosis, and treatment planning. Among various tasks, image segmentation has been one of the most important medical image analysis tasks. Recently, deep convolutional neural networks have been widely applied in medical image segmentation with state-of-the-art performance. Meanwhile, Automated Machine Learning (AutoML) has also been explored in deep neural networks, aiming to further enhance model efficiency and effectiveness. However, the existing AutoML algorithms are mainly applied on 2D network based applications (due to the computational budget), or focus on individual components of deep learning solutions (e.g., neural architecture, hyper-parameters), which could lead to sub-optimal results.
In this talk, I will take a systematic perspective, and introduce novel algorithms which automatically considers and customized most, if not all, of the components of a deep neural network-based solution for 3D medical image segmentation. The proposed algorithm can not only optimize the neural network architecture, but also estimate the hyper-parameters or other components of the model training recipes. Specifically, I will introduce new neural architecture search spaces and new AutoML algorithms to accommodate large search spaces. Experiments show that the proposed algorithms can achieve state-of-the-art performance on large-scale 3D medical image segmentation datasets.
In the rest of the talk, I will introduce the newly released MONAI project Auto3DSeg, which enables AutoML for large-scale 3D medical image segmentation. It leverages the latest advances in MONAI and GPUs to efficiently develop and deploy algorithms with state-of-the-art performance while requiring minimal user input (such as data roots and lists). The solution provides different levels of user experience for beginners and advanced researchers in the field. And it has been tested on large-scale 3D medical imaging datasets with different image modalities.
嘉宾简介
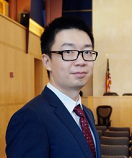
Dr. Dong Yang received his Ph.D. from Rutgers University in 2019 under the supervision of Professor Dimitris Metaxas, and has continued his research on medical image processing since then. He also interned at Siemens Healthineers (formerly Siemens Corporate Research) where he worked on several medical image segmentation projects. Currently he is an applied research scientist at NVIDIA. His research interests include medical image processing and clinical statistics. He has published in many major conferences and journals such as MICCAI, CVPR, ICCV, Medical Image Analysis, IEEE Transactions on Medical Imaging, etc.
特别感谢本次Webinar主要组织者:
叶初阳(北京理工大学)