研究中心硕士孙宇的一篇论文“Unsupervised Medical Image Feature Learning by De-Melting Reduction Auto-Encoder”被Neruo Computing刊发。
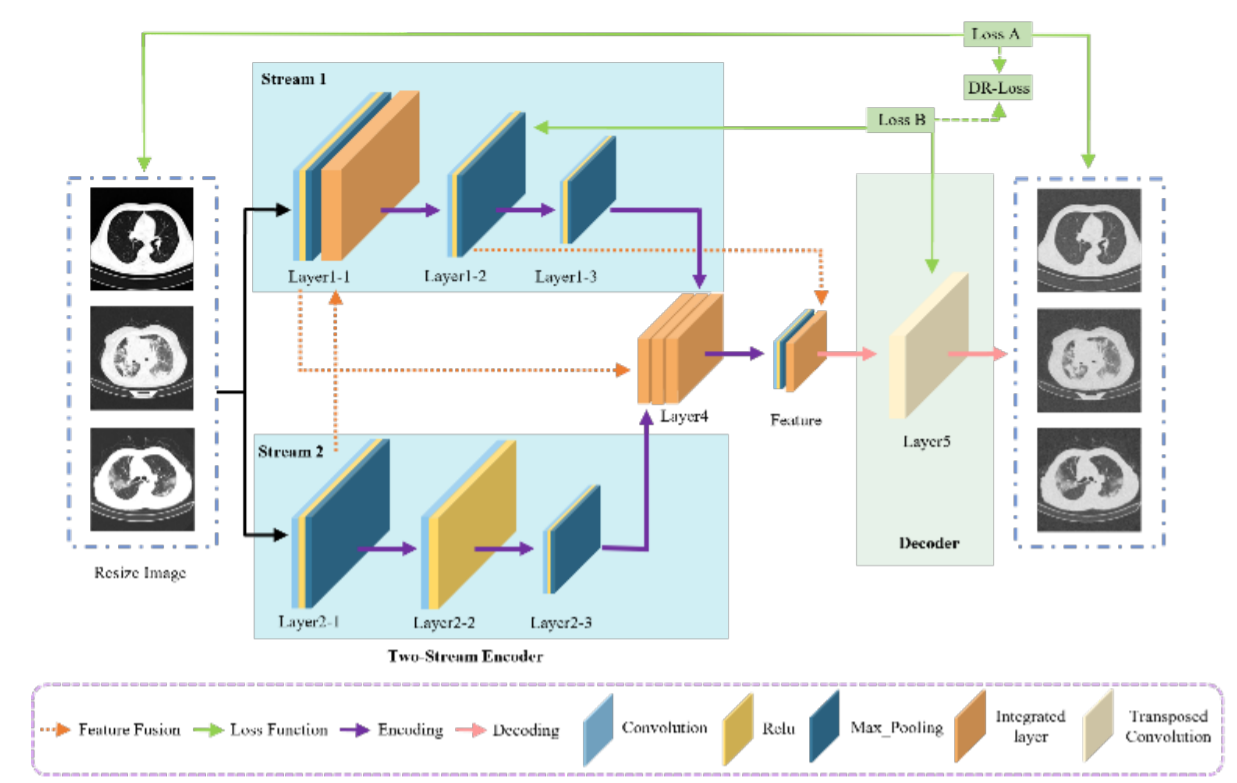
【摘要】无监督特征学习是医学图像分析中最基本、最受重视的问题之一。虽然它在医学图像分析中取得了显著的进步,但由于其特征表达能力弱、模型学习效率低、鲁棒性差,仍然具有挑战性。针对上述不足,本文提出了一种新的无监督特征学习方法--融减自动编码器(MRAE)。更具体地说,构建了一种联合融合网络结构,该网络结构不仅可以改善目标特征的表达,而且可以减少特征解码和参数的损失。为了获得稳健的解,设计了一种新的分解重构损失函数来加强相邻特征提取器层之间的语义上下文,成功地避免了单一优化目标的模型学习能力不足,提高了提取特征的质量。最后,在400名受试者的乳腺超声图像和6000名受试者的肺部CT图像上进行了广泛的实验,验证了该方法的有效性。实验结果表明,DMRAE显著减少了标注工作量,并且在很大程度上优于现有的标注方法。
[Abstract]Unsupervised feature learning is one of the fundamental and highly prioritized problems during medical image analysis. Although it has made remarkable improvement in medical image analysis, it remains challenging because of weak feature expression ability, low model learning efficiency and weak robustness. To address previously mentioned deficiency, a novel unsupervised feature learning method, named De-Melting Reduction Auto-Encoder, is proposed in this paper. More specifically, a joint fusion network structure is constructed, which can not only improve the expression of target features but also reduce the loss of feature decoding and parameters. To obtain the robust solution, a newly-designed decomposed reconstructed loss function is used to strengthen the semantic context between adjacent feature extractor layers, successfully avoiding the insufficient model learning ability from the single optimization objective and improving the quality of extracted features. Eventually, extensive experiments on datasets with breast ultrasonographic images of 400 subjects and lung CT images of 6000 subjects are conducted to demonstrate the effectiveness of the proposed method. Experimental results demonstrate that the DMRAE significantly reduces the annotation effort and outperforms prior existing methods by a significant margin.