近日,研究中心硕士生侯昊的一篇论文“Semi-Cycled Generative Adversarial Networks for Real-World Face Super-Resolution”被IEEE Transactions on Image Processing(SCI一区,CCF-A类期刊,影响因子11.041)录用。(论文链接地址:https://ieeexplore.ieee.org/document/10036448)
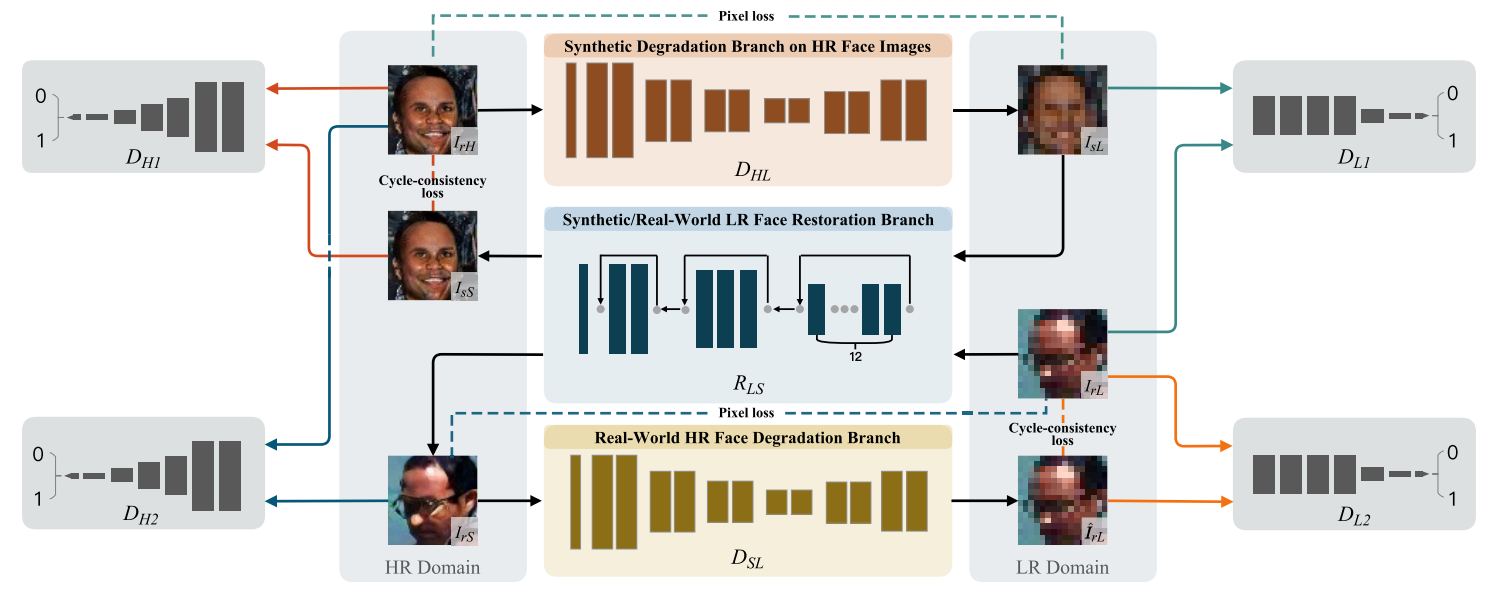
论文摘要: 真实世界的人脸超分辨率(SR)是一种高度不适定的图像恢复任务。完全循环的 Cycle-GAN 架构被广泛用于在人脸 SR 上实现有前途的性能,但在现实场景中具有挑战性的情况下容易产生伪影,因为联合参与同一退化分支会影响最终性能生成器获得的真实世界和合成 LR 之间的差距。为了更好地利用 GAN 对真实世界人脸 SR 的强大生成能力,在本文中,我们分别在前向和后向循环一致重建过程中建立两个独立的退化分支,而这两个过程共享相同的恢复分支。我们的半循环生成对抗网络 (SCGAN) 能够减轻现实世界 LR 人脸图像和合成 LR 图像之间的域差距的不利影响,并通过正则化的共享恢复分支实现准确和稳健的人脸 SR 性能通过前向和后向循环一致的学习过程。在两个合成数据集和两个真实世界数据集上进行的实验表明,我们的 SCGAN 在恢复真实世界人脸 SR 的人脸结构/细节和定量指标方面优于最先进的方法。代码将在 https://github.com/HaoHou-98/SCGAN 公开发布。
Abstract: Real-world face super-resolution (SR) is a highly ill-posed image restoration task. The fully-cycled Cycle-GAN architecture is widely employed to achieve promising performance on face SR, but is prone to produce artifacts upon challenging cases in real-world scenarios, since joint participation in the same degradation branch will impact final performance due to huge domain gap between real-world and synthetic LR ones obtained by generators. To better exploit the powerful generative capability of GAN for real-world face SR, in this paper, we establish two independent degradation branches in the forward and backward cycle-consistent reconstruction processes, respectively, while the two processes share the same restoration branch. Our Semi-Cycled Generative Adversarial Networks (SCGAN) is able to alleviate the adverse effects of the domain gap between the real-world LR face images and the synthetic LR ones, and to achieve accurate and robust face SR performance by the shared restoration branch regularized by both the forward and backward cycle-consistent learning processes. Experiments on two synthetic and two real-world datasets demonstrate that, our SCGAN outperforms the stateof-the-art methods on recovering the face structures/details and quantitative metrics for real-world face SR. The code will be publicly released at https://github.com/HaoHou-98/SCGAN.