近日,研究中心硕士生王国力的一篇论文“MRChexNet: Multi-modal bridge and relational learning for thoracic disease recognition in chest X-rays”被Mathematical Biosciences and Engineering(SCI四区,影响因子2.6)录用。(论文链接地址:https://www.aimspress.com/article/doi/10.3934/mbe.2023942)
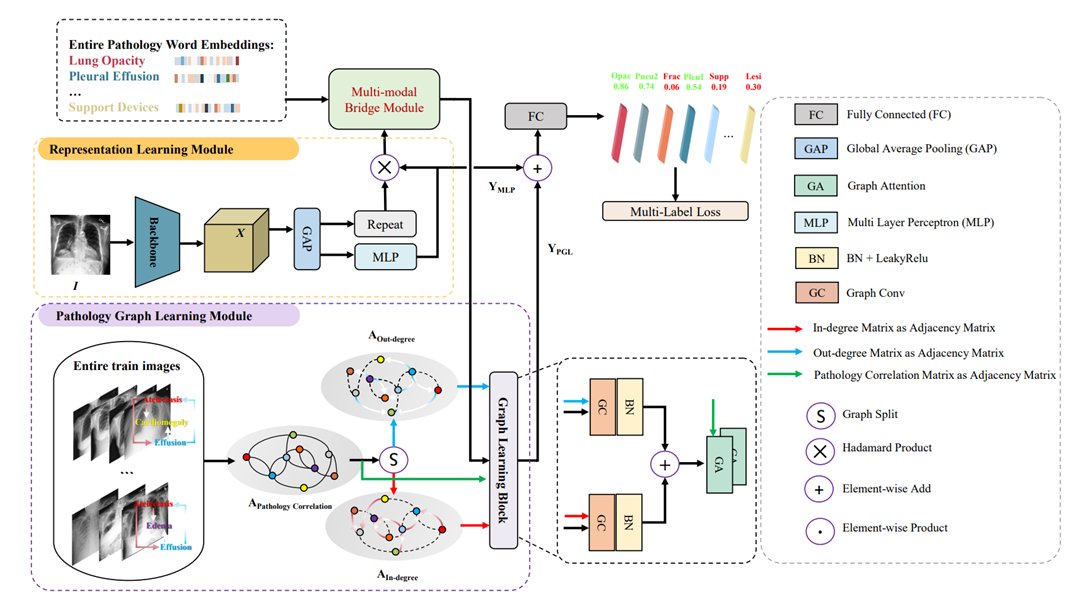
论文摘要: 在诊断胸部X射线图像(chest X-ray, CXR)中的多种病变区域时,放射科医生通常在做出最终诊断前应用疾病间的病理关系。因此,全面分析疾病之间的关系对于提高模型的识别性能至关重要。然而,大多数考虑病理关系的自动化CXR诊断方法将不同模态的数据视为独立学习对象,忽略了不同模态数据间病理关系的对齐。此外,一些使用无向图来模拟病理关系的方法忽略了图上的有向信息,难以准确模拟所有病理关系。在本文中,我们提出了一种新的多标签CXR诊断模型,称为MRChexNet,并由三个模块组成:表示学习模块、多模态桥接模块和病理图学习模块。表示学习模块捕获像素级特定疾病的病理学特征。多模态桥接模块执行不同模态数据中病理关系的跨模态对齐。病理图学习模块将疾病间的有向关系建模为有向图。最后,在病理图学习模块中设计的图学习块完成对不同模态数据中病理关系的集成学习。我们在两个大型CXR数据集(ChestX-Ray14和CheXpert)上评估了MRChexNet,并取得了最先进的性能。14种病理的平均曲线下面积得分为0.8503(ChestX-Ray14)和0.8649(CheXpert)。实验结果表明,MRChexNet能有效地对齐不同模态数据中的病理关系,并学习到病理间更精准的关联。与现有方法相比,MRChexNet具有高准确性和强泛化能力。MRChexNet可以为自动化CXR诊断识别做出贡献。
Abstract: While diagnosing multiple lesion regions in chest X-ray (CXR) images, radiologists usually apply pathological relationships in medicine before making decisions. Therefore, a comprehensive analysis of labeling relationships in different data modes is essential to improve the recognition performance of the model. However, most automated CXR diagnostic methods that consider pathological relationships treat different data modalities as independent learning objects, ignoring the alignment of pathological relationships among different data modalities. In addition, some methods that use undirected graphs to model pathological relationships ignore the directed information, making it difficult to model all pathological relationships accurately. In this paper, we propose a novel multi-label CXR classification model called MRChexNet that consists of three modules: a representation learning module (RLM), a multi-modal bridge module (MBM) and a pathology graph learning module (PGL). RLM captures specific pathological features at the image level. MBM performs cross-modal alignment of pathology relationships in different data modalities. PGL models directed relationships between disease occurrences as directed graphs. Finally, the designed graph learning block in PGL performs the integrated learning of pathology relationships in different data modalities. We evaluated MRChexNet on two large-scale CXR datasets (ChestX-Ray14 and CheXpert) and achieved state-of-the-art performance. The mean area under the curve (AUC) scores for the 14 pathologies were 0.8503 (ChestX-Ray14) and 0.8649 (CheXpert). MRChexNet effectively aligns pathology relationships in different modalities and learns more detailed correlations between pathologies. It demonstrates high accuracy and generalization compared to competing approaches. MRChexNet can contribute to thoracic disease recognition in CXR.