近日,研究中心硕士生任钰、王国力的一篇论文“MM-SFENet: Multi-scale Multi-task Localization and Classification of Bladder Cancer in MRI with Spatial Feature Encoder Network”被Physics in Medicine and Biology(SCI三区,影响因子3.5)录用。(论文链接地址:https://iopscience.iop.org/article/10.1088/1361-6560/ad1548)
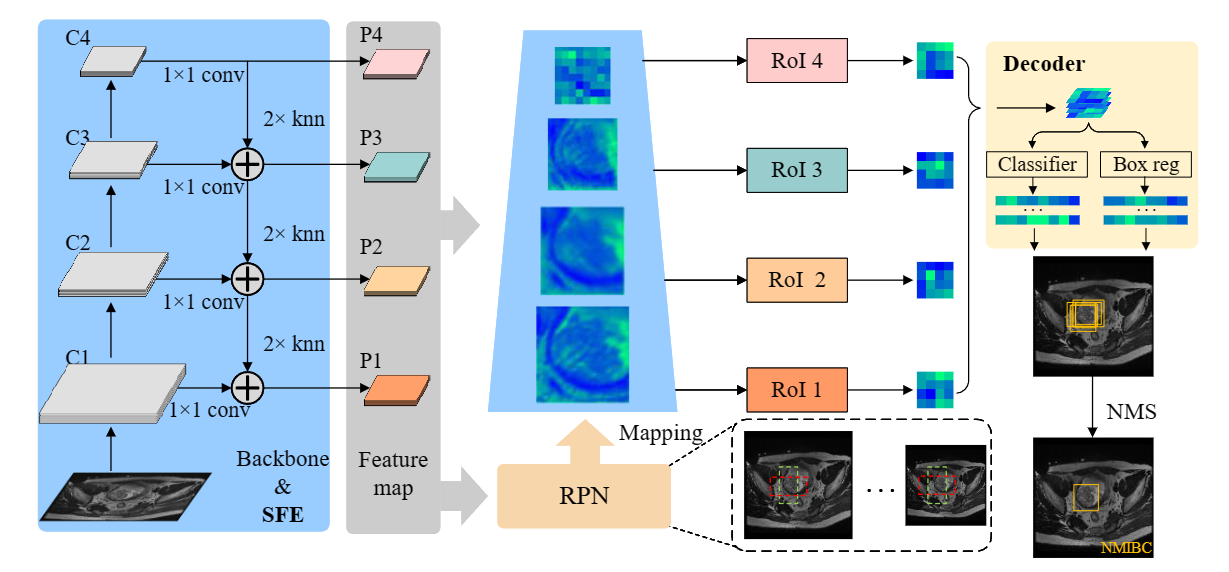
论文摘要: 目标:膀胱癌症是一种常见的恶性泌尿系肿瘤,其主要亚型为肌肉浸润性和非肌肉浸润性。本文旨在实现基于MRI的癌症侵袭性自动定位和分类。方法:与以往分割膀胱壁和肿瘤的工作不同,我们根据肿瘤和膀胱壁之间的空间关系的分类标准,提出了一种新的端到端多尺度多任务空间特征编码网络(MM-SFENet),用于对癌症进行定位和分类。首先,我们构建了一个带有残余块的主干,以区分膀胱壁和肿瘤;然后,设计了一个空间特征编码器来对骨干的多层次特征进行编码,以学习准则。实验结果:我们用IoU损失代替Smooth-L1损失进行多任务学习,以提高分类任务的准确性。通过学习从医院癌症患者收集的两个数据集,使用mAP、IoU、Acc、Sen和Spec作为评估指标。试验结果在试验集1上可达到93.34%、83.16%、85.65%、81.51%、89.23%,在试验集2上可达到80.21%、75.43%、79.52%、71.87%、77.86%。意义:实验结果证明了所提出的MM-SFENet在膀胱癌症定位和分类方面的有效性。为癌症的分期提供了一种有效的辅助诊断方法。
Abstract: Objective. Bladder cancer is a common malignant urinary carcinoma, with muscle-invasive and non-muscle-invasive as its two major subtypes. This paper aims to achieve automated bladder cancer invasiveness localization and classification based on MRI. Approach. Different from previous efforts that segment bladder wall and tumor, we propose a novel end-to-end multi-scale multi-task spatial feature encoder network (MM-SFENet) for locating and classifying bladder cancer, according to the classification criteria of the spatial relationship between the tumor and bladder wall. First, we built a backbone with residual blocks to distinguish bladder wall and tumor; then, a spatial feature encoder is designed to encode the multi-level features of the backbone to learn the criteria. Main Results. We substitute Smooth-L1 Loss with IoU Loss for multi-task learning, to improve the accuracy of the classification task. By learning two datasets collected from bladder cancer patients at the hospital, the mAP, IoU, Acc, Sen and Spec are used as the evaluation metrics. The experimental result could reach 93.34%, 83.16%, 85.65%, 81.51%, 89.23% on test set1 and 80.21%, 75.43%, 79.52%, 71.87%, 77.86% on test set2. Significance. The experimental result demonstrates the effectiveness of the proposed MM-SFENet on the localization and classification of bladder cancer. It may provide an effective supplementary diagnosis method for bladder cancer staging.